RESEARCH PROJECTS
Data Driven Approach to Approximation, Counting and Mechanism Design (D2ALGO)
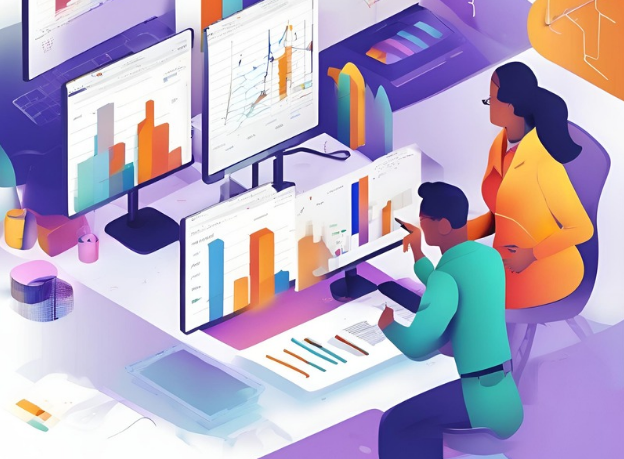
The proposed research lies in the general research direction of “beyond worst-case analysis” in the Theory of Algorithms, and aims to investigate how (and to which extent) the design of algorithms (mostly approximation and online algorithms, sampling and counting algorithms and truthful mechanisms for public and private good allocation) can benefit from the recent advances in Computational Learning Theory and Machine Learning.
LEAP: A Deep-Learning Framework Towards an Efficient and Adaptive AI Engine for Visual Perception
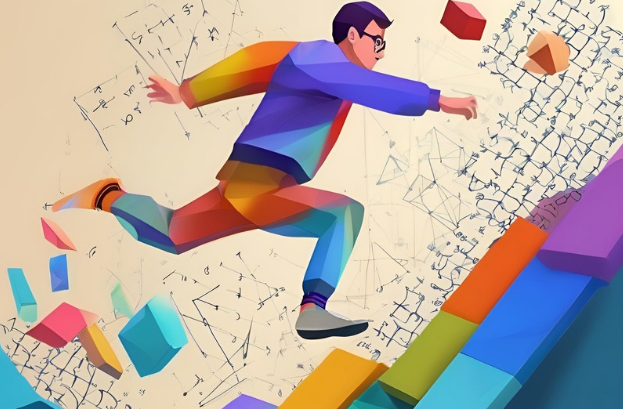
The LEAP project aims to lay out the algorithmic and mathematical foundations of a new framework for visual learning that harnesses the power of deep neural networks to develop the next generation of unsupervised, few-shot learning and continual learning approaches in the visual domain.
Learning in Multi-agent RL: Going beyond two-player zero-sum stochastic games
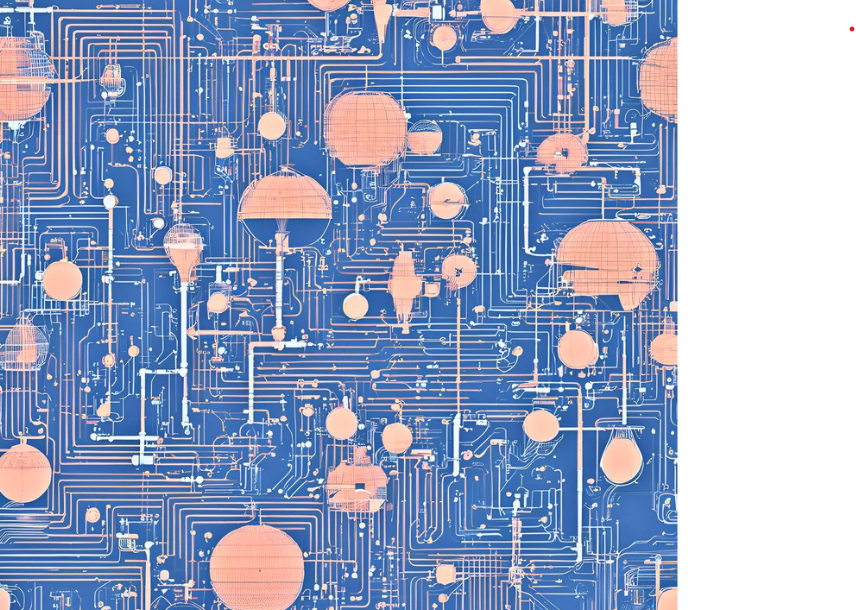
Reinforcement Learning (RL) has been a fundamental driver for recent advances in Artificial Intelligence, ranging from super-human performance in games including Go and Starcraft to decision making, autonomous driving and robotics.
AI-Enabled Digital Pathology for Precision Oncology
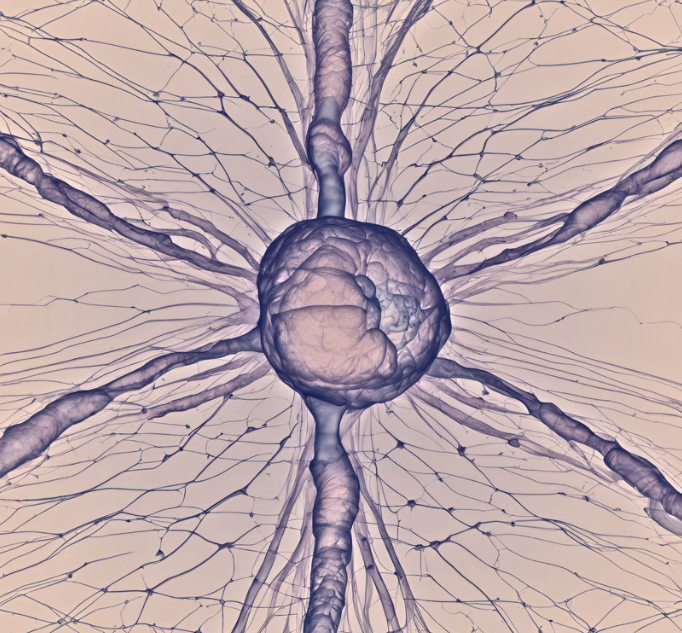
Artificial Intelligence has become the pivotal research and innovation vehicle in health care.