Learning in Multi-agent RL: Going beyond two-player zero-sum stochastic games
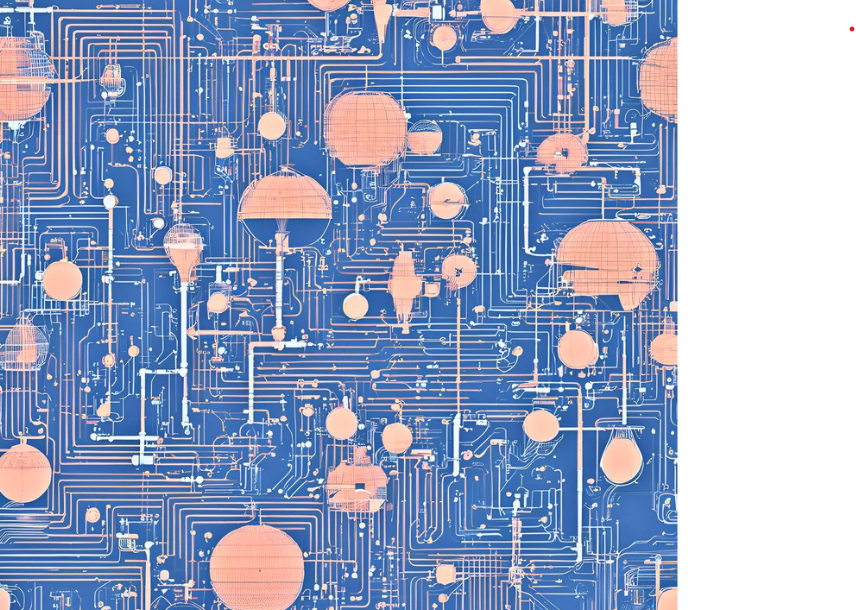
Reinforcement Learning (RL) has been a fundamental driver for recent advances in Artificial Intelligence, ranging from super-human performance in games including Go and Starcraft to decision making, autonomous driving and robotics.
A core ingredient behind the success of single-agent RL systems, which are typically modelled as Markov Decision Processes (MDPs), is that an optimal stationary policy always exists and moreover, there exist efficient algorithms that provably converge to it. Recent progress has also been made towards understanding Multi-agent RL (MARL) settings with two players in which the reward of one player is the negation of the reward of the other player, i.e., zero-sum stochastic games.However, in practice, a majority of the systems involve multi-agent interactions, going beyond single-agent and two-player zero-sum settings. In such cases, despite the notable empirical advancements, there is a lack of understanding of the theoretical convergence guarantees of existing MARL dynamics. The aim of this proposal is to investigate MARL settings in which convergence to Nash policies - one of the most common solution concepts in MARL - can be provably guaranteed, exploiting techniques from Dynamical Systems, no-regret learning and non-convex optimization, pushing forward our preliminary findings in [Leonardos et al 2022, Fox et al 2022]