Data Driven Approach to Approximation, Counting and Mechanism Design (D2ALGO)
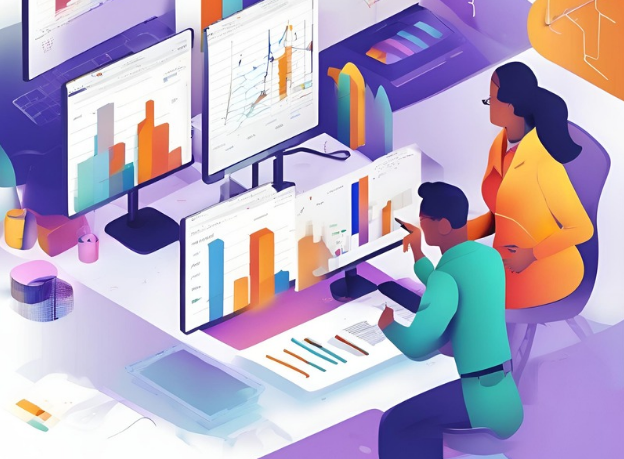
The proposed research lies in the general research direction of “beyond worst-case analysis” in the Theory of Algorithms, and aims to investigate how (and to which extent) the design of algorithms (mostly approximation and online algorithms, sampling and counting algorithms and truthful mechanisms for public and private good allocation) can benefit from the recent advances in Computational Learning Theory and Machine Learning.
We will apply the recently developed framework of universal learning to data-driven algorithms, aiming at more refined and distribution-dependent learning rates for specific problems and particular classes of algorithms. Moreover, we aim to develop a principled approach to the design of non-adaptive and partially adaptive data-driven algorithms and truthful mechanisms based on the notion of universal approximation for combinatorial optimization problems. We will also investigate whether a principled and general approach, which combines best possible worst-case guarantees with the information available about the input distribution, allows for the design of near optimal learning-augmented algorithms. As for approximate counting and sampling, we will investigate whether learning techniques can improve the convergence rate of random walks, by fine-tuning transition probabilities of the underlying Markov chains, and whether learning techniques can be used to efficiently identify counting dichotomies.