FairGraph: Mitigating and Explaining Unfairness in Graphs
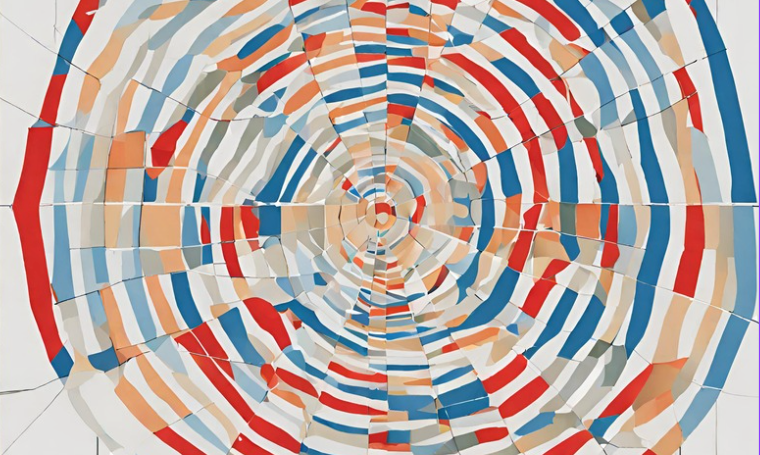
Various data-driven algorithmic systems are being used to assist or even replace human decision making, thus raising various concerns regarding the trustworthiness and ethical standing of such systems.
Algorithmic fairness attempts to conceptualize and operationalize notions of fairness through formal technical definitions, metrics, and mitigation methods. The FairGraph team will address fundamental problems of algorithmic fairness in graphs. Research will be along three axes. The first axis involves understanding and formalizing the conditions that lead to unfairness as graphs evolve over time as well as intervening though link recommendations to make the network fairer. The second axis is about handling representation bias in graph collection and sampling. The third axis is about explaining unfairness by providing counterfactual-based explanations for the causes of unfairness. A fourth action is dedicated to educational and awareness activities related to fairness in AI.