Studying Multilingual Neural Models with Symbolic Approaches
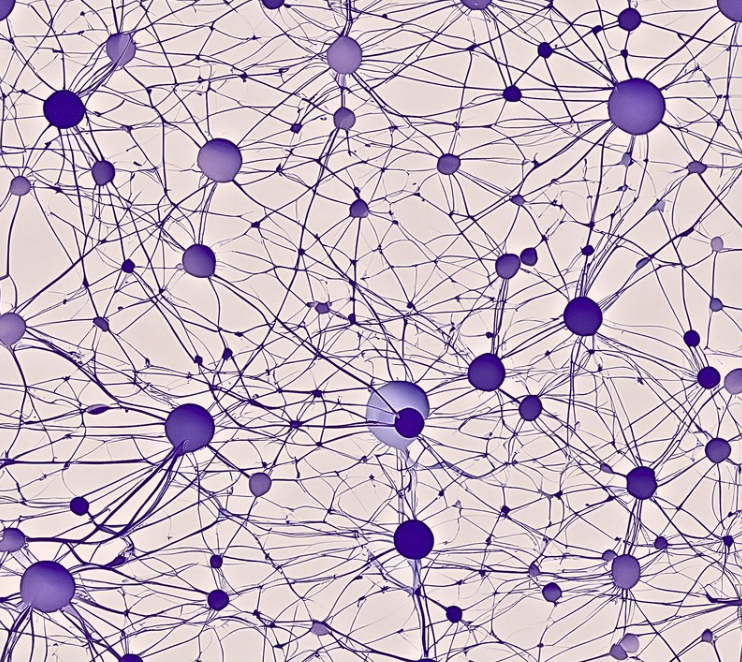
Despite the notable success of neural multilingual languages models (MLMs) in language AI, their internal mechanics remain quite unclear; they simply train models on data from as many languages as possible.
The community lacks answers to crucial questions: How is each language and their interactions modeled internally? Which linguistic phenomena are represented, and why? What facilitates cross-lingual transfer? What type of biases do languages rich in data exert on the representation of less-resourced languages and dialects? And, no language is a monolith; within the same language variation results from sources such as regional, social class and mode-of-usage differences. MLMs have largely ignored language variation because they rely on large amounts of data, which only few standardised widely spoken languages can provide. By treating less resourced varieties as noise, they neglect both the scientific evidence they encapsulate and the millions of their speakers. We will turn to symbolic approaches to understand the inner workings of large MLMs. New insights will guide our effort to inject linguistic knowledge into neural models, aiming to learn how to profit from human expertise and work with sparse data. We will work with multiple low-resource language varieties, particularly the severely technologically under-served Greek ones.