Learning-oriented Approaches in Optimization, Fair Division and Mechanism Design
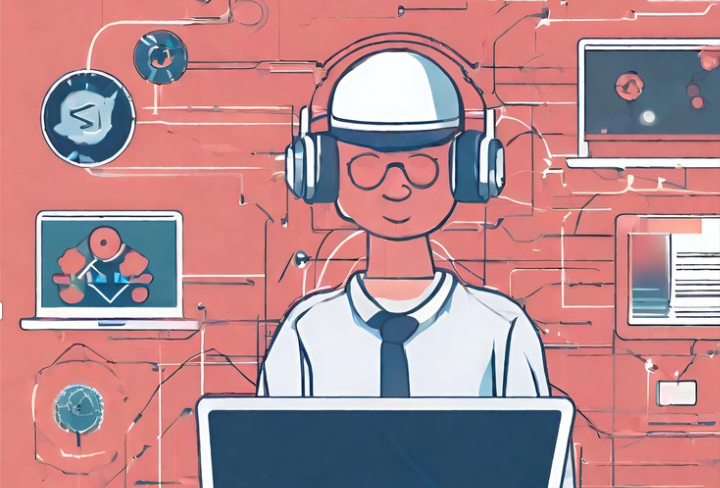
The focus of this project is on incorporating learning-oriented perspectives in the context of optimization, fair division and mechanism design.
To this end, we plan to work on three main directions and emerging challenges along this front. The first one concerns the design of learning algorithms for min-max optimization problems. Such problems are equivalent to finding Nash equilibria in zero-sum games and find several applications in machine learning, especially with regard to training neural networks. Our second direction is to consider resource allocation problems with the goal of achieving fairness criteria. This has been a very active area in the last decade but with several algorithmic questions remaining still unresolved. These questions become even more intriguing in models with uncertainty or when the input parameters are revealed in an online fashion. Finally, the last pillar of our project evolves around mechanism design, i.e., the design of algorithms in the presence of strategic agents. For this we plan to consider data-driven paradigms, where the algorithm designer can take into account data of past instances to come up with (possibly erroneous) predictions on parameters of the underlying problem. The goal therefore will be to take into account such learning-augmented scenarios, in order to obtain auction mechanisms with improved performance guarantees.