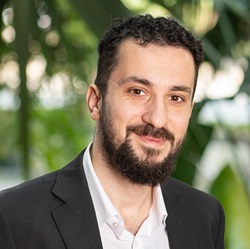
Emmanouil Vasileios Vlatakis Gkaragkounis
Assistant Professor
University of Wisconsin–Madison, USA
Short Bio:
Emmanouil V. Vlatakis Gkaragkounis (Manolis Vlatakis) is an Assistant Professor at the University of Wisconsin-Madison in the Department of Computer Sciences. He received his PhD degree from Columbia University in the city of New York. He was a Postdoctoral Researcher at the Simons Institute for Theory of Computing. He has published over 20 research papers in high-impact CS & ML journals and conferences. His research focuses on bridging the gap between theory and practice, particularly in the context of multi-agent AI systems. For example, he investigates how different agents, such as autonomous vehicles or trading algorithms, interact with one another when each is driven by its own objectives. These interactions can lead to both unexpected successes and significant challenges. Manolis aims to develop a theoretical framework that explains why certain modern heuristics—widely used techniques or rules of thumb—perform exceptionally well in some scenarios but fail in others. This framework could provide valuable insights into optimizing decision-making processes in environments where multiple agents with diverse incentives coexist, such as in competitive markets or collaborative robotic teams. |