SPIEL: Statistics- and Physics-Inspired methodologies for Equilibrium Learning
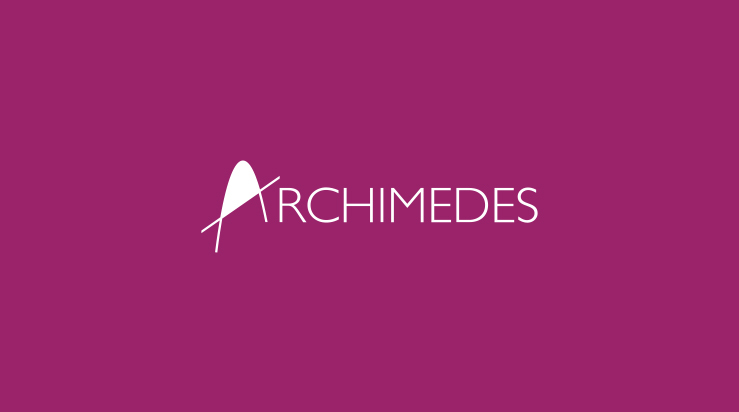
Despite the recent spectacular successes of artificial intelligence (AI) – from generative models to self-driving cars – the machine learning (ML) algorithms that power them remain poorly understood, especially when interacting with each other.
By necessity, the training of interacting ML models breaks away from “learning as usual” loss minimization problems, and must rely instead on the theory of min-max optimization and multi-agent equilibrium learning. Unfortunately however, recent results have shown that training an AI architecture with two or more networks can be exponentially more difficult than training a single one.In view of this, our proposal aims to understand how the training gap between single- and multi-agent ML models can be bridged in certain contexts, and what types of behavior to expect when it cannot. Specifically, we intend to provide a statistical characterization of the models that arise in multi-agent AI architectures, to quantify the learning distance between single- and multi-agent models, and to design efficient training methods that transcend the gradient- based paradigm. Our proposal seeks to address these questions with a blend of statistical physics and differential geometric techniques which dovetail naturally with existing toolboxes from optimization and game theory.