Safe and Reliable ML for Decision Making through Cryptographic Primitives
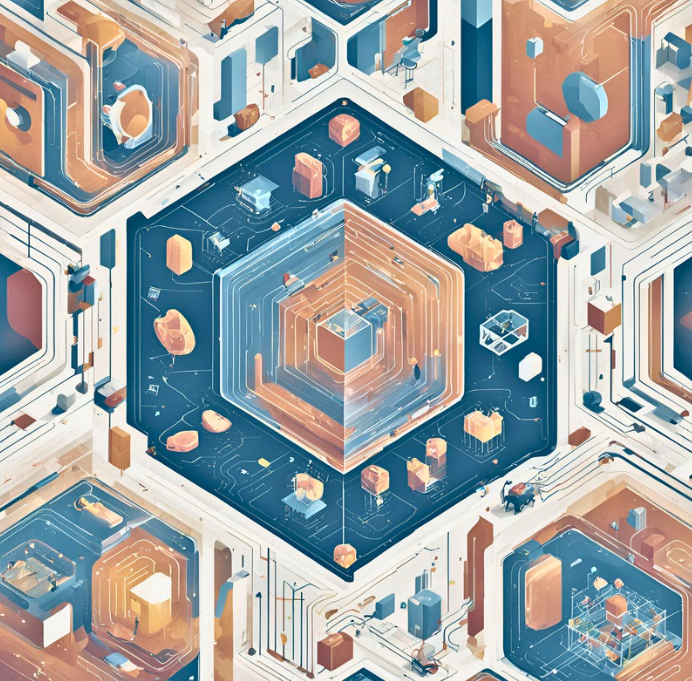
The success of Machine Learning (ML) has led to a surge of automated systems for consequential decision- making: loan approvals, medical diagnoses, and probation decisions can all be made through an ML system nowadays.
The significance of these decisions and the sensitive nature of users' data create tensions between the organization that deploys the ML algorithm and the users regarding the safety and reliability of the decision- making process. Once people understand how an ML-based system works, they often try to strategically alter their data to obtain better outcomes for themselves (either by gaming the system or by achieving recourse). Although keeping the ML algorithms secret from the users may obstruct gaming, it concurrently disallows the bona fide amelioration of users' features and creates questions about the reliability of the ML algorithm. In this research agenda, we put forth models that combine Incentive-Aware ML and Cryptography to address the aforementioned tensions. We will investigate how cryptographic tools, such as zero-knowledge proofs, allow an organization to provide formal recourse guarantees about the decision-making procedure while keeping all other information secret. Central to our study will be the tradeoff between accuracy, interpretability, and privacy that naturally arises when ML is used for decision making.