Principled Methods for Classification with Noisy Data
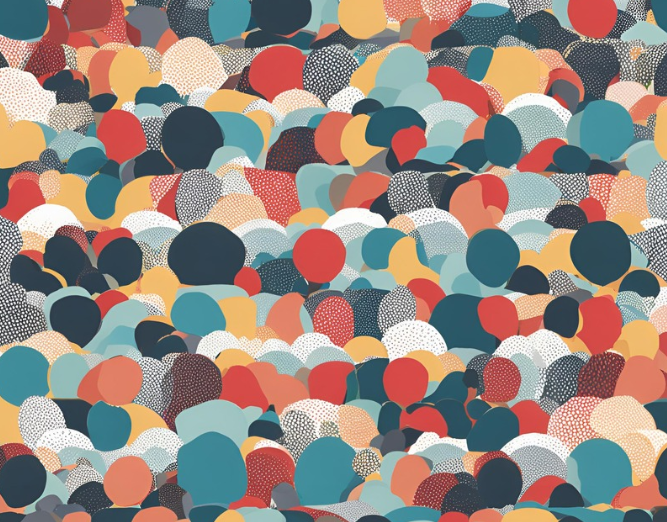
Learning with noisy data is an important research challenge in both theory and practice.
It has been repeatedly observed that a small amount of noise can significantly hinder the performance of the ML models as errors in the training data tend to propagate in the models predictions. Yet, noise is quite widespread in many tasks and is an important bottleneck in applying ML techniques in many scientific domains. The project aims to develop novel computationally efficient methods for learning with noisy data. The work will focus on robust classification under semi-random label noise. The work will build on a large line of recent work, including work of the LPI, that focuses almost in its entirety on binary classification to extend the theory to the multiclass case. The multiclass case presents novel difficulties not present in the binary case and all known efficient methods in the literature fail to extend. An important focus of the project is in developing practically relevant algorithms that are grounded on the theoretical results and are competitive with state of the art approaches.