Latent Markov Decision Processes and Reinforcement Learning
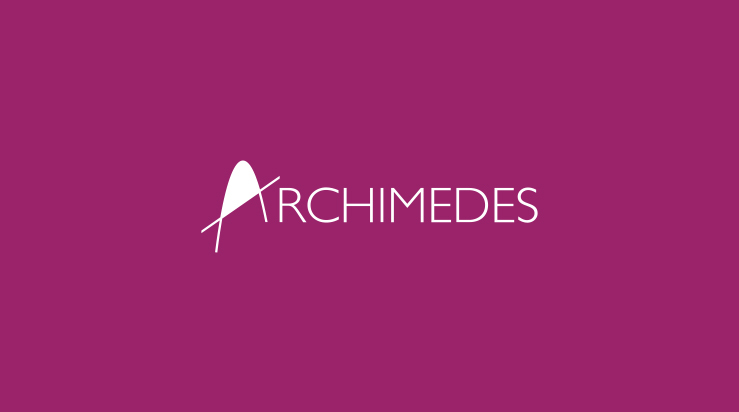
We propose to study sequential decision making problems where the environment is unknown, and moreover, where the environment can only be partially observed.
As we explain below, partial observation is in general the death knell for sequential decision-making problems. Here, we are interested in problems where the unobserved part of the environment changes slowly, or not at all. This setting abounds in important practical problems, from autonomous driving, AI and medicine, to e-commerce and beyond. Many other important problems share this special structure. Though, as we discuss, little is known about such latent partially observable problems, our recent results suggest that this is a theoretically rich area. Because of the importance and applicability of the latent model achievability and impossibility results in this area can have significant impact.