Equilibrium Computation and Learning in Multi-Agent Systems
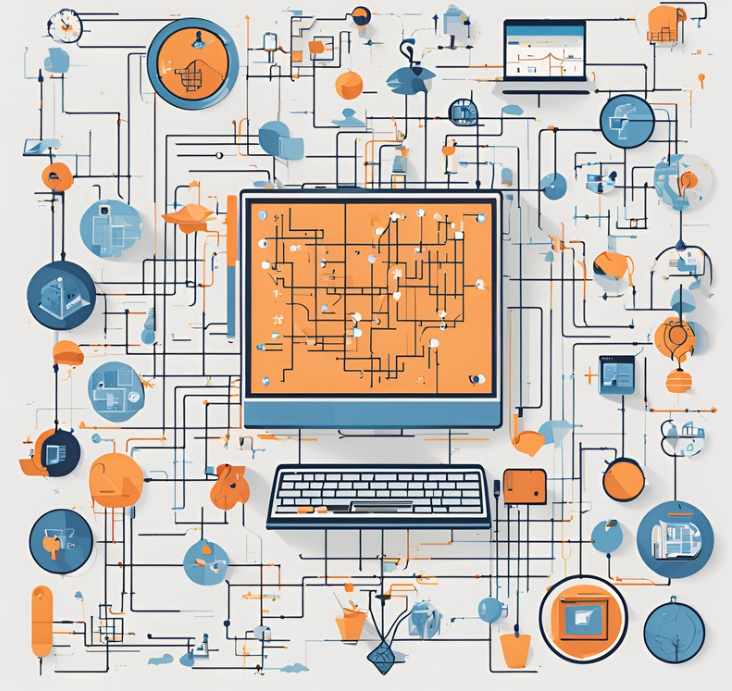
Machine Learning has witnessed tremendous success in various applications in the past decade.
A particularly successful paradigm is to formulate the training of the machine learning system as minimizing a single loss function and solve it via some efficient optimization algorithm. Many emerging applications of machine learning start deviating from this single objective viewpoint and are better captured as a game between multiple intelligent agents/algorithms. In this proposal, we plan to investigate the following question: What are the meaningful solution concepts in machine learning systems, and when can they be realistically computed? The questions we propose concern min-max optimization in normal form games, equilibrium computation for multi- player stochastic games, and new and alternative solution concepts.