Artificial Intelligence (AI) through Network Systems Neuroscience: Developing AI architectures based on biological principles for guiding experiments in visual cortex
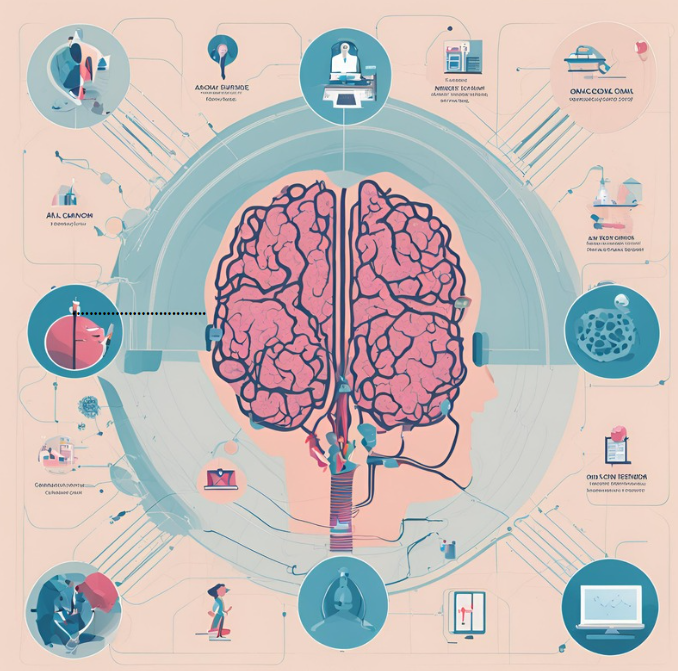
NeuroAILab examines AI from the perspective of network/systems neuroscience, aiming to develop ANN- architectures based on biological principles and then further guide experiments in visual cortex.
It focuses on the role of the spontaneous-conditions in encoding the visual-stimuli and behavioral states, and predict the functional-connectivity under visual presentations from the spontaneous functional-connectivity. We will then integrate our findings in the development of unsupervised or self-supervised learning architectures that employ time-series, memory, and multi-graph-structures (e.g., using VAEs), training them using the extensive biological datasets. The identification of the “principal” features of these architectures, as learnt through the latent representation, is an important aspect. Metrics, such as robustness, joint information-transfer and energy- efficiency, and training size will be considered. We will also develop SNN-variants in which the connectivity is guided by our findings in biological networks. Its innovative aspects include the use of rich biological datasets, the incorporation of our findings in the design of such architectures, and the graph-similarity algorithms/metrics. The potential long-term impact is not restricted in biology but can help us better understand computationally- efficient architectures: how the brain’s massively interconnected neuronal-networks make robust inferences about the world from limited data and in noisy environments, exceeding the performance of current ML algorithms.