Machine Learning and Natural Language Processing
DESCRIPTION
Natural Language Processing has seen important advances over the past decade. While deep learning models have achieved impressive performance, even demonstrating common-sense knowledge, they are commonly viewed as uninterpretable black-boxes. We combine deep learning with deductive reasoning techniques from the symbolic Artificial Intelligence tradition, to produce new algorithms capable of reaching conclusions requiring multiple inference steps, using premises and conclusions expressed in natural language. We are also interested in understanding multilingual language models. While neural multilingual language models (MLMs) have notable success, their internal mechanics remain quite unclear. We explore symbolic approaches to understand the inner workings of large MLMs and investigate how to inject linguistic knowledge into neural models, aiming to learn how to benefit from human expertise and work with sparse data.
RESEARCHERS
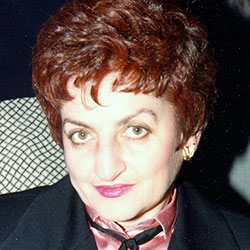
Angela Ralli
University of Patras
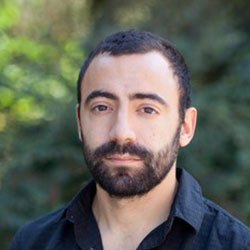
Antonios Anastasopoulos
GEORGE MASON UNIVERSITY
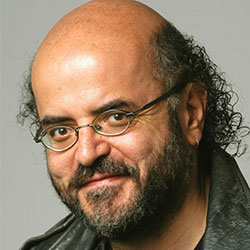
Christos H. Papadimitriou
Columbia University
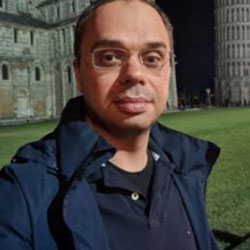
Dimitris Galanis
Research Center 'Athena'
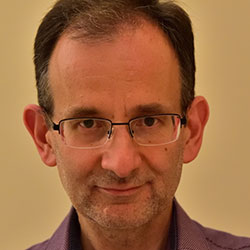
Ion (Ioannis) Androutsopoulos
Athens University of Economics and Business
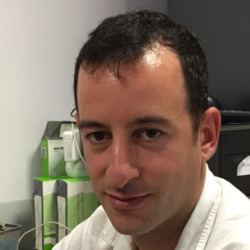
John Pavlopoulos
Αthens University of Economics and Business
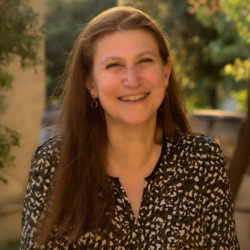
Sophia Ananiadou
University of Manchester
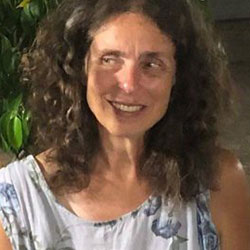
Stella Markantonatou
Research Center Athena
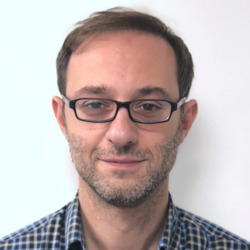
Themos Stafylakis
Athens University of Economics and Business
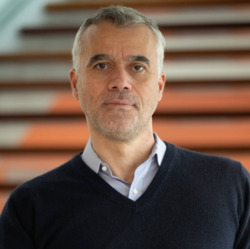